General
Revolutionizing drug discovery with AI

Summary
Do you know what penicillin (an antibiotic), chloral hydrate (a sedative), warfarin (an anticoagulant), and iproniazid (an antidepressant) have in common? They are all drugs developed in the early 20th century. And they were all discovered by accident.
Unlike in the 19th and the early 20th century, most drugs today follow a sophisticated development process.
But some things are still the same.
Even today a drug takes 12-15 years and $1B+ to hit the market since its conception. Even after all this hefty time and cost investment, only 11% of drugs hit the market; the remaining 89% are killed in the lab because of safety concerns or efficacy issues with the drug, or because of the economic infeasibility of further development.
After reading the above paragraph, it is very natural to ask questions like:
Why is the time and cost of investment so high? Why is the probability of success so low? Can we not turn this around?
After all high investment and low success rates translate to high drug prices that are borne by us, the patients.
There just might be an answer.
Artificial intelligence might be the panacea for this rather inefficient process.
But before we go deeper into the how, it is important to understand the what and the why — What do we need for a drug to work? And why is such a huge investment required for taking the drug from the lab to the market?
If you are well-acquainted with basic concepts of pharmacology, skip to the Emerging Trends section.
If you are hearing the term pharmacology for the first time, we recommend going through the entire blog (psst, it is not rocket science!).
What? Drugs, Leads, Targets, Biomarkers, and 8-ball pool
We need two things for a drug to work — the drug itself, also known as the lead (as in lead candidate) and a site in the human system for the lead to bind, also known as the target. Although not necessary, having a third thing, called the biomarker, can drastically improve the chances of success (up to 6x). The biomarker is used by scientists to check if the drug is working in the desired manner or not. Implying that it is any measurable molecule in the human system whose quantity changes in response to the lead. Glucose is an example of a biomarker for diabetes.
Let's take an example of 8-ball pool here:
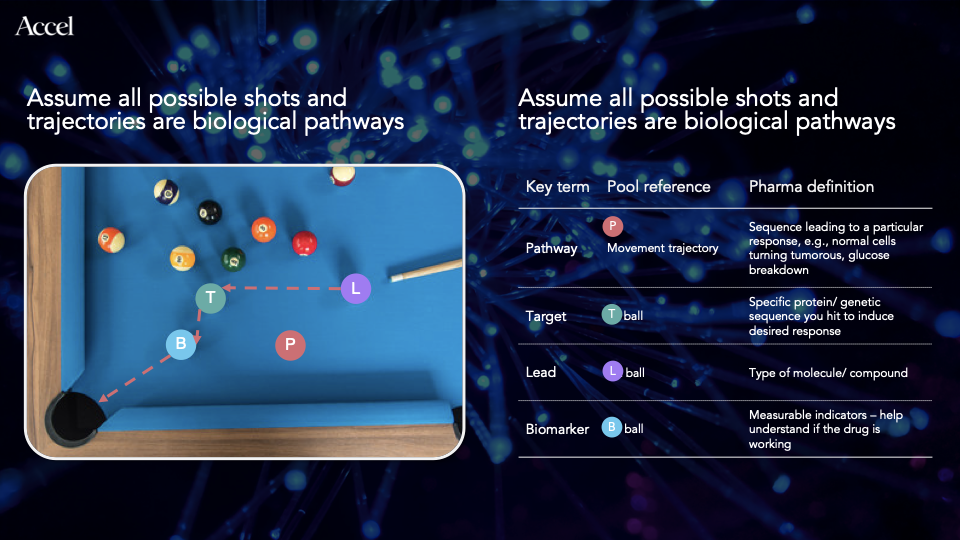
Refer to the right hand side of the infographic above to understand biology through an analogy of 8-ball pool. This image helps explain the commonly used terms in drug discovery and development process.
Why? Experiments, Mechanism of Action, and Economic Viability
A de novo drug is an unknown substance that can potentially be lethal if the exact mechanism of action is not understood properly, thereby, requiring a comprehensive process. Let's have a closer look at what it takes for a drug to hit the market.
The process starts with experiments on cell lines/ patient xenografts. Once the mechanism of action is established with a sufficient degree of confidence, the different dosage of identified lead candidates is administered to animals, before finally conducting clinical trials on humans.
.png)
We need to internalize four notable features of the drug discovery and development process to appreciate the need for computational models:
1. Each step of the drug discovery process is more expensive, more intensive, and closer to real-life conditions than the last
Results can vary largely from one step to the next and the development might need to be terminated at any step
2. The process prioritizes establishing safety over efficacy (needless to explain why), implying that without establishing safety, we cannot establish the efficacy
We do not know if the drug actually works or not until really late in the process
3. For truly novel findings, we do not know/ have the right biological models for experimentation
We cannot conclusively say if our findings are accurate
4. It is a highly iterative process: A new set of experimentations are run for every hypothesis, meaning, to validate a new drug combination, all experiments need to be re-run
Costs escalate quickly with every new hypothesis
In a nutshell, the drug discovery and development process becomes more complex and elaborate as it progresses, with an inherent risk of failure at each step, and we cannot be sure about it before actually running the experiments. And we need to run individual experiments for each hypothesis or target-lead candidate combination.
Given that each experiment costs several thousand to a hundred thousand dollars, and to conclusively understand the mechanism of action and identify real insights, one needs to run several hundred to thousand experiments.
If the scientific sanctity of the process is maintained, each project will end up burning multiple billion dollars only in the first step of the process.
Furthermore, for most of the truly novel discoveries, we do not have the right cell lines and transgenic animal models.
We are living in the future, the future of drug discovery
High costs, long development time, and unavailability of the right models make a strong case for computer simulation-driven drug discovery. So, finally let's understand the how.
How can computers run these experiments?
Like any other artificial intelligence model, drug discovery simulations require robust real-world patient data sets for training the algorithms. These data sets consist of longitudinal patient data, including clinician's notes, diagnostic reports (imaging, pathology, biopsy, genomics, proteomics), and response reports to various types of treatments administered.
As we know, this data is sensitive information and resides with hospitals, pharma cos., and academic institutions, we need algorithms that can not only take away relevant insight but also algorithms that can de-identify (or anonymize) the patient data and provide a secure network for sharing these insights, while not moving the raw data from the owner's servers.
.png)
We, at Accel, take a value chain view to classify the tech companies in the drug discovery space, and here are the three broad categories:
1. RaaS/ SaaS (Report/ Software as a Service)
This category includes two sub-categories — novel diagnostics and novel digital data generation companies and software for managing patient data and other research data for institutions. This is the starting point for computational drug discovery, generating and digitizing real-world patient data.
2. DaaS (Data as a Service)
Companies in this category provide a secure network to enable sharing sensitive, real-world patient data from data sources (hospitals, research institutes, and in some cases pharma cos.) to data users (pharma cos., research institutes). These companies act as a bridge between owners of data and users of data.
3. IaaS (Insights as a Service)
Companies in this category use real-world data, publicly available data from sources, such as TCGA, Human Atlas, UniProt, etc, and self-generated data at scale to deliver insights relevant for developing novel therapeutics. Since this category is the core of the drug discovery process/ value chain, let's double click on this category to explore some emerging trends.
Computationally discovered targets and digitally designed drugs
From a scientific perspective, there are two aspects of a drug discovery process — biology and chemistry. Biology helps us understand the exact mechanism of action and thereby come up with targets and biomarkers. While chemistry comes into the picture during the drug candidate design phase.
Here is a brief infographic summarizing various types of computational drug discovery technologies.
.png)
Computational Biology
The two sub-categories within computational biology rely on public data sources and self-generated proprietary data at scale. Modern biological methods, such as iPSC (induced Pluripotent Stem Cells), CRISPR gene editing system, etc. modern machine learning approaches (convolutional neural network algorithms are used most commonly) are employed.
Although using similar setup, approaches, and methods, there is a huge difference in the types of algorithms being deployed. Hence, two sub-categories. These two sub-categories are inspired by the two methods conventionally deployed by pharma cos. in the drug discovery phase — namely, TDD (Targeted Drug Discovery) and PDD (Phenotypic Drug Discovery).
Systems biology simulations work on the principles of targeted drug discovery, which is a hypothesis-driven method of coming up with targets, biomarkers, and leads. The computational approach involves representing cellular signaling pathways as graphs/ CNNs and these networks/ relationships are developed by leveraging existing research literature on cellular biological pathways and by generating biological data at scale.
High throughput phenotypic screening works on the principle of phenotypic drug discovery, which is a brute force, hit-and-trial method of coming up with targets, biomarkers, and leads. The computational approach involves running experiments at scale through automation and zeroing in on cellular molecules whose regulation (removal or hyper-activation) leads to the desired outcome (tumorous cell death or cell proliferation).
Systems biology simulations, although tougher to develop and ace, are more suited from both effectiveness and adoption point of views.
System biology simulations help understand the biology or science behind the drugs in detail and in a comprehensive manner. This means that there will be no surprises in later parts of the drug development process (refer to section *Why? Experiments, Mechanism of Action, and Economic Viability* above), thereby improving chances of success. Moreover, as potential collaborators to whom novel findings are out-licensed are R&D heads, with deep scientific expertise, at big pharma cos., it is easier to convince them about the findings as there is logical and deep-scientific backing information available about the biology.
Computational Chemistry
Computational chemistry technologies vary largely depending on the type of drug candidates one is working with — small molecules, proteins, antibodies, etc.
The core idea is to create a drug molecule that binds to the identified target and leads to the desired outcome with no or manageable side effects. Side effects and outcomes to a large extent are determined by using computational biology algorithms. While the binding interaction is determined through the use of computational chemistry algorithms. Artificial intelligence helps in increasing the diversity of candidate molecules by simulating millions and billions of potential chemical/ biological combinations (chemical in case of small molecules, biological or amino acids in case of proteins). Of course, running millions and billions of experiments is not feasible. This increase in simulations enables the discovery/ design of the most appropriate lead candidate or the set of most promising lead candidates.
As you might have realized, this thesis is a primer to artificial intelligence in medicine, we will not go into details of the algorithms. If you are interested in learning more about algorithms, you can read about AtomNet from Atomwise and Centaur Chemist from Exscientia for small molecules, and AlphaFold from DeepMind for protein design algorithms.
How do these companies make money? The business of drug discovery
At early stages, AI in medicine companies typically partner with mid-large pharma cos. to further build and validate tech stack. Contract sizes for such partnerships are typically in the range of a few hundred thousand dollars. Real value inflection comes once the company has worked for 1-2 years on ~5 small projects (typically 3-6 months each), and built their own proprietary asset pipeline.
These deals fetch high upfront payments, ranging from one to ten million dollars with subsequent milestone-based payments, which can go as high as single-digit billion dollars based on the market potential for the asset.
Here is a table summarizing business models/ partnership models with big pharma cos. for AI in medicine companies:
.png)
In recent times, three key business models have emerged in the biopharma space, these models differ on two aspects:
- Asset offering to big pharma companies — target vs lead, and
- Partnership model — one-time asset handover vs asset co-development
In business models 1 and 2, novel targets are out licensed to the development partner/ pharma company, while in model 3, optimized leads are out licensed. The fundamental difference between models 1 and 2 is the level of engagement with the development partner during the remainder of the validation process. Model 1 is a sales model as opposed to co-development as in model 2.
On the right hand side of the illustration above, you can see one example of a deal on each of the three models. As you can see, there are four key components of the deal structure:
- Upfront payment — the amount of sum which is received at the time of signing the deal,
- Equity investment — the capital for a certain ownership in the company,
- Milestone-based payment — payments made over the development lifetime of the asset, from pre-clinics to clinics
- Royalties — a percentage of sales when the asset hits the market
There is another non-monetary benefit of partnering for drug discovery companies — years of experimental data, which early-stage startups can leverage to further refine their algorithms.
Please reach out to us at ra@accel.com and sdang@accel.com if you are building a tech company in medicine or would like to hear more thoughts on the space from us or just brainstorm with us.
Researched and written with Siddhant Dang, analyst at Accel.
We’re looking for the next generation of successful Indian marketplaces. If you’re an early-stage marketplace founder, apply now here or learn more about the #DecodingMarketplaces Startup Hunt.
We’d love to hear about your experiences with marketplaces. Let us share our learnings and build a better and stronger ecosystem. Write to us at seedtoscale@accel.com to be a part of the Accel family.
Subscribe to SeedToScale
/subscribe to get the latest stories from SeedToScale/